Behind the scenes of Klaviyo AI—with the people who built it
As new AI tools roll out nearly every day, it can be tempting to prioritise learning and implementing them as quickly as possible.
But how did they get built? Why did they get built? What’s the human need behind them?
If you wonder about what drives a certain tool’s creation, or the intricacies behind their development, Klaviyo’s Data Science Podcast is for you.
The episodes give you the breakdown on how the team here at Klaviyo:
- Sees research as creative
- Focuses on learning as a career-long habit
- Achieves a profound understanding of the data before they build—and keeps learning as they build
- Seeks feedback from multiple perspectives
“We do our best work by sharing knowledge,” says Mike Galli, senior data scientist at Klaviyo. “Everybody has a unique perspective, and each of those unique perspectives could be the one that opens up a brand-new door in terms of project planning.”
“Failing isn’t failure,” adds Annika Sougstad, data scientist II at Klaviyo. “If you stay focused on having a good process, the results will come and you’ll be a little less afraid of failure—because failure is learning. Being able to think outside the box is super invaluable.”
If you stay focused on having a good process, the results will come and you’ll be a little less afraid of failure—because failure is learning. Being able to think outside the box is super invaluable.
Keep reading to learn how the Klaviyo data science team thinks outside the box when creating and iterating our AI tools.
How generative AI can unlock the email inbox with the right subject line
“Not every key opens every door, right? You have to actually craft your key so it opens a particular door,” says Michael Lawson, data science manager at Klaviyo.
That’s the metaphor Klaviyo’s data science team used to visualise the importance of subject lines—and get machine learning right so it complements and elevates a marketer’s efforts to create the perfect marketing email.
“For a lot of brands, the huge set of constraints around subject lines are really intimidating and become an optimisation problem,” says Royce Yap, a machine learning expert and former data scientist at Klaviyo.
For a lot of brands, the huge set of constraints around subject lines are really intimidating and become an optimisation problem.
Klaviyo’s data science team knows the process of coming up with a good subject line is actually quite complex.
So, they built 3 sub-features to give marketers the flexibility and agency to own their subject line creation:
- Generate: solves writer’s block by helping users generate fresh subject lines based on their inputs
- Analyse: see top performing subject lines and understand what works best for your audience
- Learn: teaches marketers best practices, as well as providing examples of stellar subject lines
Listen to Episode 24: Changing the subject (line).
How Klaviyo’s NLP humanises customer service chats
As a consumer yourself, how many times have you found yourself in a conversation with a brand about customer support and something about their responses just feels…off?
Klaviyo’s data science team sought to solve this problem through natural language processing (NLP). They knew that canned responses are an expression of how long it takes customer service reps to manually type out more original, authentic conversations.
So, their SMS AI to reply to texts makes sure that suggested messages are personalised based on every business’s tone. (Not like typical chatbot messages, where you feel like you’re talking to a robot.)
How did they do it? Smit Kiri, senior ML engineer at Klaviyo, shares in the episode that the team had to “manually go through a lot of data to be able to find a pattern and think about a new intent.”
The process they use is so effective and unique, they’ve actually filed a patent for it.
Listen to Episode 27: NLP conversations at scale.
How the team uses AI to calculate surprise factors
Every November, ahead of BFCM, Klaviyo’s data science team dedicates themselves to understanding how brands can prepare for the unexpected.
Most recently, they built an anomaly detection feature that automatically spots issues with flows in every Klaviyo customer’s account. The model learns from past patterns of a metric to determine if something looks broken, or if the flow isn’t getting the desired engagement for another reason.
Their biggest challenge?
“We don’t have the truth about whether things are anomalies or not, so the process for developing these models is to label a set of graphs with what we think of as anomalies,” says Lilia Staszel, senior full stack data scientist at Klaviyo.
Check out the podcast to hear how customers of varying sizes get the guidance they need quickly, with a system flexible enough to accommodate every type of customer and flow.
Listen to Episode 29: Detecting the unexpected.
How speed in AI implementation met the end of open rates—and created a soft landing for brands
Apple’s iOS 15 update presented an intractable problem for brands—the effort to ensure email privacy protection made it nearly impossible to trust open rates. How could the team at Klaviyo build Smart Send Time—the feature that helps you figure out the time your subscribers are most likely to open your emails—and make sure it gives accurate recommendations?
To succeed, they leaned on their expertise—and creativity. They even had lead data scientist Ian Delbridge pivot to focus on Smart Send Time exclusively.
Delbridge and the data science team:
- Correctly randomised each experiment, which rendered Smart Send Time somewhat immune to the iOS update
- Excluded machine opens in guided warming, and quickly too, which meant Klaviyo didn’t have a broken product as the update rolled out
- Mitigated the problem of guided warming under iOS 15 by adjusting thresholds based on observed changes in global and regional changes
Listen to episode 32: How iOS 15 changed the world (and data science answered).
Helping marketers better understand customers—and get data they can actually interpret
Klaviyo’s data science team was motivated to satisfy two deep needs brands share: to understand customers and serve their needs better, and to save time doing it. They built Klaviyo CDP’s RFM analysis tool to tackle both.
RFM—recency, frequency, and monetary value—analysis refers to the grouping of customers based on their purchase behaviour—specifically, how recently they made a purchase, how frequently they purchase, and how much they spend.
And it’s great for determining marketing strategies because it’s “something brands can wrap their heads around to describe the current state of a customer,” says Nick Hartman, engineering manager at Klaviyo. “It’s very interpretable, not a black box.”
RFM is great for determining marketing strategies because it’s very interpretable, not a black box.
Listen to the conversation to learn:
- What product and technical challenges the team ran into
- How they score customers with respect to recency, frequency, and monetary value (hint: it’s not as simple as it seems, especially for scaled scores)
- Which of the 3 required the most creative thinking to assign scores
Listen to episode 42: Unlocking customer insights with RFM.
Get behind the scenes access every month
AI is hurtling forward quickly—but it’s been in Klaviyo’s DNA for years. Klaviyo’s Data Science Podcast has been live since 2020, and it’s a great behind-the-scenes look at how best-in-class engineers build AI tools for the future.
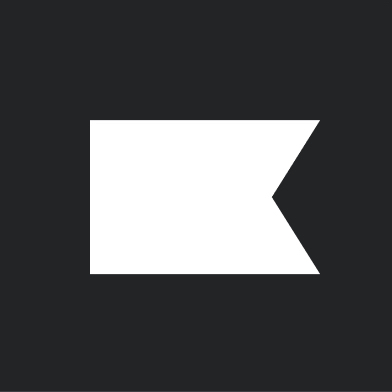
Related content
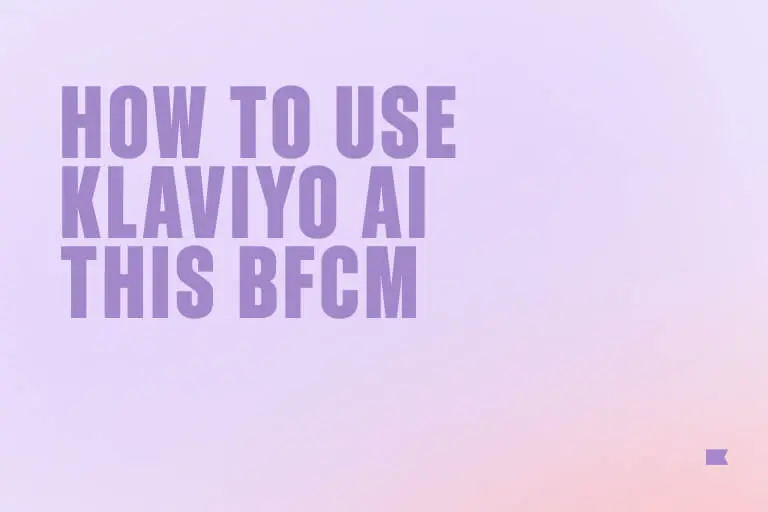
Streamline your holiday season with time-saving AI features that generate flows and segments, optimise forms, and get campaigns to the right person at the right time.
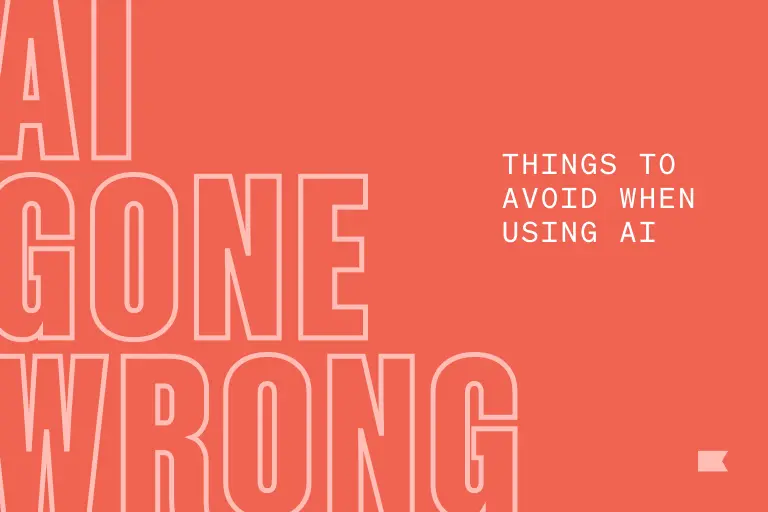
Discover the common AI pitfalls for ecommerce businesses and customer relationships—and how to fix them.
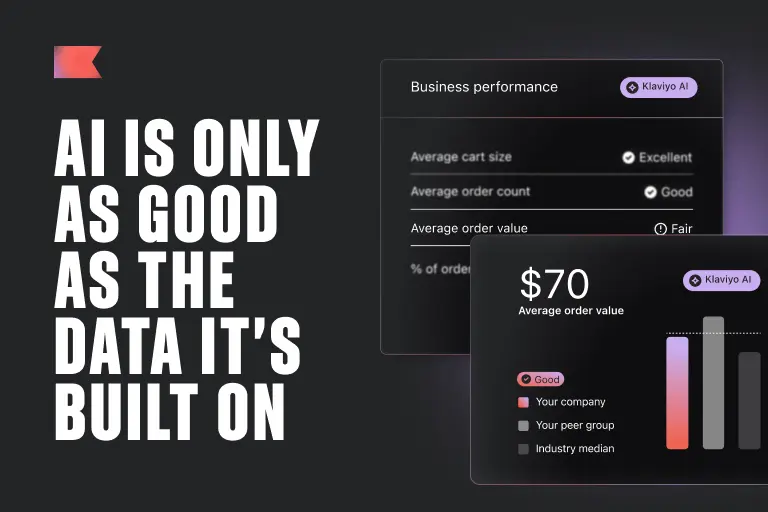
Learn why clean customer data is integral to the development of artificial intelligence and machine learning in martech platforms.